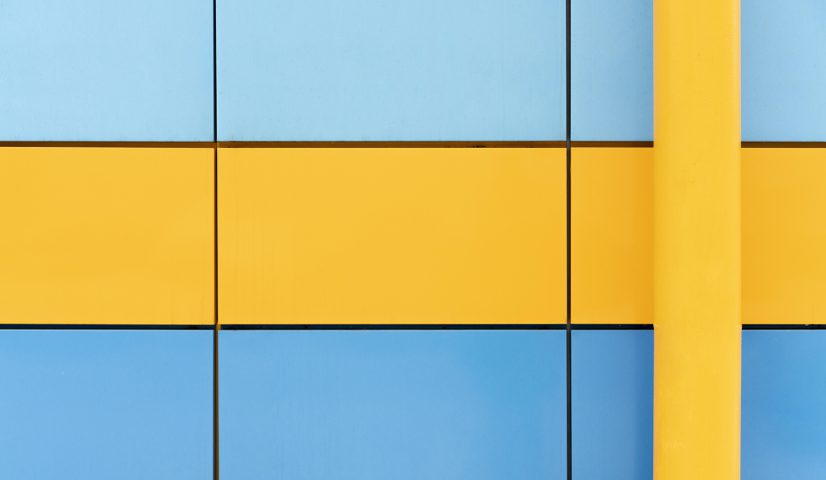
A Better View of Performance
If I had to speculate, I’d venture that every Nonprofit Operator worldwide wrestles with Attribution at least a few times a week. At least I hope it’s not just me. The intricacies of some Attribution is truly perplexing. There’s always a piece of the puzzle that doesn’t quite fit. And which model to adopt…
- Is the last click more significant than the first click?
- How do you factor in frequency?
- Why should I place faith in Google’s “Data-Driven Attribution” when its inner workings remain a mystery?
- And what’s the deal with the fact that “Facebook doesn’t share data with Google Analytics and you need to decide how much you trust the platform?”
I struggle to trust any of them. But, I get it, no framework is without its flaws.
Brand Awareness metrics, for instance, are ineffective when viewed in isolation. Every report I’ve encountered invariably emphasizes the campaign’s success in driving a “5x increase in ad recall and 3x increase in consideration,” yet this never translates into a noticeable uptick in donation volume.
MMM is slow. And I’ve yet to witness any Org fully utilizing it to inform all its spending decisions due to limited use cases. And so on for every other model.
Measuring digital performance is undeniably challenging. The industry’s current frenzy over the deprecation of third-party cookies has held measurement and attribution in the spotlight – but does it truly alter the landscape, or are we merely swapping one enigma for another?
What do you mean you haven’t figured out measurement yet?!
I don’t think anybody has figured it out. Below I’ve dropped my “summary framework”. There’s no perfect model to answer every “what works and what doesn’t?” question, and any of them used alone is no better than a coin flip – otherwise, everybody would be using it already.
What I’ve shared below is how I used various performance views throughout the donor lifecycle – each contributing to an overall picture for me and others in the Org. Perhaps this is helpful to you too? None of them require expensive, long-to-implement tools and should apply to Orgs of all sizes.
One note before we jump in: Most measurement frameworks are geared towards determining Channel, Ad, or Creative performance – not Audience performance. I discussed segmentation and finding the most likely-to-convert donors in SPN #15. Here I’ve focused on measuring Channel performance in reaching those audiences at various stages of the lifecycle. There’s no such thing as “Cost per Donation in Display” or “Cost per Donation in Search” – there’s only “Cost per Donation for Audience A in Display.”
A Summary Framework for Each Step of the “Funnel”
1. Journey Stage: Top of Funnel
Measure: Generating new, first-time donors
Key questions for this lifecycle phase: What/Who is the best audience? What is the right channel mix/budget for each channel to reach that audience?
How: Incrementality Testing and Geo Holdouts worked best for me.
When launching a new channel – or twice a year for channels that have been running for a long time already – this approach helped me:
- Pulling the Geo Performance report at a ZIP code level for the given channel.
- Separating them into 3 even segments based on spend to reflect Scale.
- Within each quartile, ranking ZIP codes from best to worst based on the revenue generated, ROI, and one more qualitative metric – I usually use Average Donation Value or Conversion Rate.
- Creating a summarized, “final” rating as a sum of 3 ratings above.
- Within each of the 3 segments, separating ZIP codes into 4 quartiles based on this ranking
- Randomly picking 3 out of 12 resulting segments – and turning off the specific channel spend for half of the ZIP codes for a month.
I usually look at what I call the “dynamic control group” – for the month when media is turned off, monitoring the MoM metrics for the affected ZIP codes (is revenue going down? Is my count of donations decreasing?) versus the same MoM metrics for the non-affected ZIP codes in the same segment, comparing not the actual values but their change. This approach has continuously helped me make sense of whether each of the channels I’m running is contributing to the performance of campaigns and whether that contribution justifies continuous investment.
Also, Brand Awareness measured as an Increase in Branded Paid Search Terms is another one of my favorite metrics for the top-of-funnel channels. Google Analytics path-to-conversion report is immensely helpful here, showing whether exposure to any of the channels is followed by a Branded Paid Search impression.
- For Branding campaigns, I’m pulling those numbers into an aggregated table, looking at the “cost of generated paid search impression” and then using that to compare branding channels or campaigns against one another.
2. Journey Stage: Mid-Funnel
Measure: Converting one-time donors to recurring
Key question: Which channel (campaign, or ad) contributes the most to moving donors to the next stage of the engagement?
How: Measurement needs to focus on Conversion Rate Lift. Conversion Paths report in GA again comes in handy.
For every new channel – and every 6 months for channels running already – run a “ghost holdout test” as the following:
- In GA, export all the conversion paths for the last month for a given audience (if I didn’t have good audience definitions, then I selected ZIP code blocks, same as I described in Step 1) – separating the ones including and excluding the specific channel I’m analyzing.
- Export all these paths into the Excel file – there is an option in the GA export dropdown which immediately creates an easy-to-read spreadsheet with every touchpoint in its own cell and every path in its own row.
- Duplicate the spreadsheet and filter one version to include the pathways with the channel we are analyzing/filter another one to exclude them.
- For the version of the file that includes the target touchpoints, create two more copies
- In one of them, include only the paths that “start with” the target touchpoint – ie, they were the first click
- In the second one, include only the paths that “end with” the target touch points – ie, they were the last click
- In the third version, exclude both scenarios above – ie, the target touchpoints were only anywhere in the middle
- With 4 resulting files, you can compare the Conversion Rate to the baseline – to the paths excluding the analyzed channel – to immediately see if spending money in it makes sense, what is the incremental lift in CVR it drives, and which attribution model (First Click, Last Click, or Linear) should be used for daily optimizations without deeper analysis.
3. Journey Stage: Bottom of Funnel
Measure: Increasing the LTV of recurring donors
Key question: Does spending money in a particular channel lower churn?
How: Conversion pathways are a great resource here again, with a slight change in logic
The process is two-fold: The first step is to pull all the pathways for donors who have churned in the last period (I usually run these reports every 3 months) versus the ones who haven’t.
Count how many pathways a channel appears in a churned donors bucket and convert that to percentage – versus the same count for not churned donors’ pathways.
- For example, if the channel is Paid Search, then:
- “Paid Search -> Paid Search -> Organic” pathway should be counted once
- “Organic -> Paid Search -> Display” pathway should be counted once
- “Organic -> Display -> Paid Social” pathways shouldn’t be counted at all
That difference in percentage is a trustworthy source to calculate how much each channel is decreasing average churn rate. That decrease rate can be further used to count the “cost to not lose a donor” – counted as average cost per touch in the channel, divided by the percentage decrease.
Comparing channels by the cost to not lose a donor helps identify channels worth spending on vs the ones that can be disabled.
Wrapping Up
The above measurement “plays” are not a replacement for a holistic, one-size-fits-all attribution model or MMM – they’re too labor-intensive to use every day, or even every week. But for Orgs looking to outperform the competition, MMM or Attribution simply don’t make the cut as the only way to gauge performance. I hope the above approaches help you to test faster, improve performance, and/or have a better view at performance rather than just a coin flip.